AI Meets Biology: How AI and Evolutionary Relationships in Nature Drive Biological Discovery
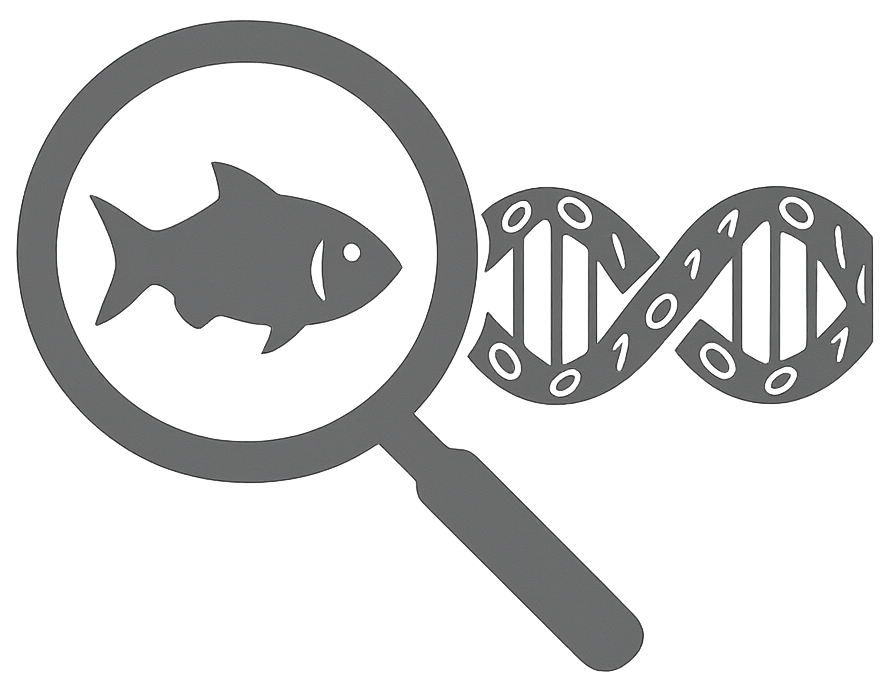
Have you ever wondered how computers might untangle the incredible complexity of nature? Two of my recent studies explore this question by embedding structured knowledge—like the relationships between species or their evolutionary history—into AI models. The proposed approaches show how understanding the interconnectedness of nature can make AI a more insightful and scientifically valuable tool in biological research, helping us tackle the challenge of extracting meaningful patterns from biological images in innovative ways.
The first study introduces the Hierarchy-Guided Neural Network (HGNN), a novel AI model that brings data and biological wisdom together to improve species classification. Unlike conventional machine learning models, which treat each species as an isolated entity, HGNN is informed by the relationships between species and their genera. While traditional machine learning models excel at recognizing patterns, they often ignore such hierarchical relationships between species—information crucial for interpreting biological data. HGNN changes this by embedding taxonomic knowledge directly into its learning process. It doesn’t just see a fish as an isolated entity but understands its place within a genus and species hierarchy. This approach boosts accuracy, especially when data is scarce, and enhances robustness to real-world challenges like occlusions and imperfections in images.
The second study builds on this idea but focuses on scientific discovery rather than classification. Using a method called Phylogeny-Guided Neural Networks (Phylo-NN), I extend AI’s capabilities from classification to discovery by using phylogenetic trees—representations of evolutionary relationships—to identify traits encoded in biological images. The model generates “Imageomes,” sequences of visual features that correspond to evolutionary signals at varying levels of ancestry. These Imageomes could enable a range of applications, from generating plausible images of ancestral species to visualizing how traits evolve over time. By incorporating evolutionary relationships, this work expands the potential for AI to contribute to biological discovery in ways that go beyond traditional methods.
These studies reflect my belief that AI can be more than a data-processing tool—it can be a catalyst for discovery when it reflects the natural structure of the world. By grounding AI in the relationships that shape life on Earth, we can create models that not only classify organisms but also deepen our understanding of their evolution and diversity. These innovations are a promising step forward in using AI not just as a tool for analysis, but as a means of advancing our understanding of the natural world. Where might this fusion of AI and graph knowledge lead us next?